Assimilation of soil moisture and leaf are index data from satellite remote sensing to improve the forecasting power of crop models
Hossein Zare
University of Hohenheim, Institute for Soil Science and Land Evaluation
Challenges
Crop yield prediction and assessment of the water used in agriculture are significant components of sustainable agriculture and water management. Although advanced crop models have been developed to simulate yield and crop water use, there is still a significant uncertainty in the prediction.
Leaf area index and soil moisture are two important state variables for vegetation processes as well as the models. These data can be estimated using satellite images during growing period. An accurate estimation of both variables and constraining the model to these data through a process of data assimilation (DA) has the potential to improve the predictive power of crop models and to reduce model uncertainty. Briefly, sequential DA methods update a modelled state variable whenever an observation (e.g., from satellite or other sensors) is available by weighting model and observation errors.
Objectives
The goal of this research project is to set up a particle filtering DA chain to sequentially improve the estimation of water fluxes and yields simulated by GECROS and NOAHMP-GECROS over the vegetation period, and analyze the related uncertainties at different scale. Objectives are:
- To evaluate the effect of DA chains (LAI-DA and Soil moisture-DA) on wheat yield prediction in Kraichgau and Swabian Alb.
- To estimate water fluxes via DA and the coupled crop and land surface model (NOAHMP-GECROS) in agricultural systems.
- To extend the application of the developed DA to a larger scale with lower quality of input data and different degrees of uncertainties regarding yield.
Expected results
Soil moisture-DA is expected to be more effective during the early growing season than LAI-DA when vegetation cover is not fully developed. During flowering, when vegetation moisture signals interferes with soil moisture signals, LAI-DA is expected to improve the model performance. Overall, sequentially updating LAI and soil moisture are expected to have complementary effects on the yield and water flux prediction.
The efficiency of DA is expected to vary across spatial scales. At the field scale where inputs are minimum biased and the model is calibrated very well, DA may have a smaller effect on model output. At larger scale, where model inputs are less well known, DA is expected to improve the model outputs significantly.
Aims
To improve a within-season yield prediction of wheat fields using GECROS and soil moisture-DA and LAI-DA approach.
To improve the water flux simulated by NOAHMP-GECROS in wheat fields applying soil moisture-DA and LAI-DA chains.
Methods
This project plans to evaluate two DA schemes at three hierarchical scales (field, federal state and national) with different degrees of uncertainty.
a) Field scale: Sentinel-2 and Landsat 7 and 8 images will be employed to estimate LAI, and Sentinel-1 and SMAP Brightness Temperature (TB) products to estimate soil moisture. The Particle Filtering method will be set up to update LAI and soil moisture as state variables in GECROS and NOAHMP-GECROS. For the linkage between the models and satellite data, a forward operator including the mentioned models, Radiative Transfer Model (RTM) and satellite observations is will be set up. The efficiency of PF-DA will be examined once using ground truth LAI and soil moisture, then remotely sensed LAI and soil moisture.
b) State scale: The developed DA chain will be upscaled to the scale of Baden-Württemberg using administrative yield data to cross-check predictions. The model will be adapted and tested in a different condition with different sources of uncertainties.
c) National scale: DA will be set up for the major wheat production area of Iran. Climate variety, unknown cultivars and different agroecosystems (irrigated vs rainfed) are new sources of uncertainty.
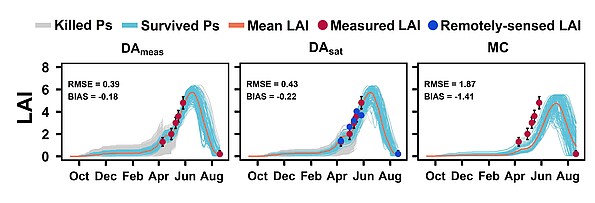
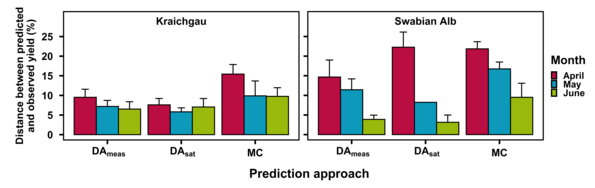